Using AI to Find High-Risk Patients for T2 Diabetes Could Save Billions and Extend Lives
Prediction model supports good healthcare and good business
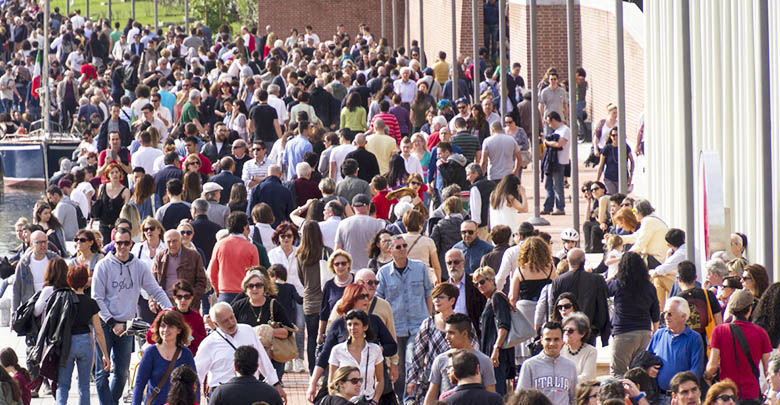
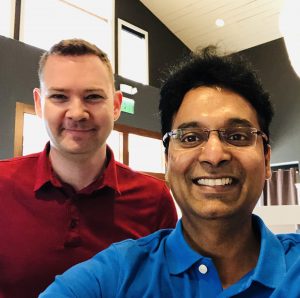
We spoke with Chaitanya Mamillapalli, MD, MRCP, FAPCR, Endocrinologist (bio) with the Springfield Clinic a 400 physician multi-specialty organization with more than 25 locations in downstate Illinois and Shaun Tonstad, Principal at Clarion Consulting about the machine learning model they developed to identify people who are at high risk of Type 2 Diabetes.
Their research study findings were presented at the 2019 American Association of Clinical Endocrinologists meeting in California and they were awarded the third place prize owing to the innovative nature of the work.
Shaun Tonstad lives with diabetes. He was diagnosed at age 9 and has lived with T1D for 30 years and his daughter was diagnosed at age 5.
Problem
Over 7 million US patients and 175 million patients globally are estimated to have undiagnosed Type 2 Diabetes. T2D may be present 4-6 years prior to a clinical diagnosis. Despite multiple guidelines, diabetes screening rates in high-risk patients are below 50% in some series. Undiagnosed T2D increases risk of diabetes-induced complications, with estimated costs near $33 billion/year in the US.
Identifying undiagnosed T2D as early as possible is an important intervention for timely treatment of hyperglycemia and decreasing the risk of diabetes complications.
Prediction Model
“Our predictive model is not aimed at diagnosing the patient but to identify high-risk patients for opportunistic screening. We wanted a reliable way to notify clinicians about those patients that should be tested for diabetes at their next visit,” says Mamillapalli.
“For a screening test, sensitivity (see definitions below) is the most important parameter. Our machine learning model demonstrates high sensitivity which is a useful metric for screening patients. Based on this sensitivity, for every 100 patients evaluated, 87 are correctly identified as being at risk for having or developing Type II Diabetes in their lifetime,” says Tonstad.
Methods
“We used nine measures that could be derived from existing electronic health records. These nine EHR measures are:
- Age
- Gender
- Race
- Body Mass Index
- Blood Pressure
- Creatinine (a measure of kidney function)
- Triglycerides (a measure of your risk of heart disease and metabolic syndrome)
- Family History of Diabetes
- Tobacco Use
“The ICD9/10 billing codes were not a reliable indicator of T2D diagnosis. Instead, for our training set of data, we classified someone as having Type 2 Diabetes if their HbA1c was 6.5% or above or their blood glucose was 140 or above,” says Tonstad.
Data Limitations
Only 14% of the 618,000 Electronic Health Records within the available dataset were complete with all nine measures. This was acceptable to develop the initial Machine Learning model and to prove the concept,” says Tonstad.
Data Integrations
The trained model will be made available to other healthcare organizations as either a cloud-based or on-premise screening tool. Data will be delivered to the model using open standards-based web services with the protection of patient privacy a core principle of the service.
Good Healthcare / Good Business
This screening approach clearly helps clinicians identify people that have or are likely to get T2D and diagnose them earlier in their disease progression. This might be implemented via health maintenance flags such as to measure HbA1c when someone comes in for a blood pressure test. This is good healthcare.
The Accountable Care Organization incents health care providers to keep their already diagnosed diabetes patients healthy. The primary measure of this health is HbA1c. If more people are diagnosed early in their T2 onset, it is likely that the HbA1c’s of these early onset people will lower the provider’s overall diabetes population average and better meet their ACO targets. This is good business.
Next Steps
They are now investigating the use of additional data points to refine and improve the accuracy of the model. However, sometimes data may be unavailable for a particular patient. “We anticipate multiple ML models running in parallel to address the differences in data available across patients,” says Tonstad.
Additional research is planned to determine if the inclusion of social determinants improves the accuracy of the model. For example, lifestyle, alcohol use, geographic area, and housing insecurity may present as risk factors. Massachusetts and other ACO organizations are starting to require that social determinants of health be addressed and our machine model can be leveraged to screen high-risk populations for chronic disease.
Although the study aimed to identify risk specifically in the absence of glycemic measures, a new model is planned that will incorporate both HbA1c and blood sugar test results for improved accuracy.
How your Provider Organization Can Participate?
“We aim to make this service available to all provider organizations so that the benefits of improved T2D screening may be broadly realized. This is a real opportunity to improve patient outcomes and lower HbA1c across managed patient populations,” says Mamillapalli.
To learn more, please email Shaun Tonstad at [email protected]. In addition to machine learning, he is an expert at integrating with and extracting data from popular electronic health record systems.
Definitions
Sensitivity
Sensitivity is an indicator of how many patients with the disease test positive.
A highly sensitive test will identify most of the patients with the disease but may also select patients without the disease. On the contrary, a highly specific test accurately identifies patients with the disease but may miss a lot of other patients who have the disease.
Screening test
A screening test is performed to find potential diseases in individuals who do not have any symptoms of the disease. The purpose is an early diagnosis of disease and by early detection effective treatment of the disease.
Screening tests are not diagnostic and will require additional testing to confirm the presence or absence of disease.
Source
Application to This Machine Learning Model
88% sensitivity: This means the machine learning model will pick 88% of the screened population and will potentially miss 12% of the patients. (This is still good compared to the traditional risk models)
68% positive predictive value: Positive predictive value is the probability that subjects with a positive screening test truly have the disease. This means that a positive test with the machine learning model indicate that the person has a 68% probability of having a diagnosis of Type 2 Diabetes.
Sources
Building Toward a Population-Based Approach to Diabetes Screening and Prevention for US Adults — https://link.springer.com/article/10.1007%2Fs11892-018-1090-5
Development And Validation of a Machine Learning Model to Predict Diabetes Mellitus Diagnoses in a Multi-Specialty Clinical Setting — AACE Poster Presentation — https://am.aace.com/2019/posters/AACE/SplitViewer.asp?PID=Mzk4MzQxNzIyMjU
The Economic Burden of Elevated Blood Glucose Levels in 2017: Diagnosed and Undiagnosed Diabetes, Gestational Diabetes, and Prediabetes — http://care.diabetesjournals.org/content/early/2019/04/01/dc18-1226
ADA Statistics About Diabetes — http://www.diabetes.org/diabetes-basics/statistics/
The Importance of β-Cell Failure in the Development and Progression of Type 2 Diabetes — https://academic.oup.com/jcem/article/86/9/4047/2848331